In this essay, I'll attempt to simplify such portentous omens by first prying open physics and then neuroscience.
The 20th century atomic physicist, Ernest Rutherford is famous for having said, "That which is not physics is stamp collecting". Let's indulge Rutherford for now, as we try to understand what he meant by physics. But first, let's start with a brief history of studying planetary motion.
Stamp collecting
Tycho Brahe, the Danish astronomer is most famous in the popular imagination for having observed the first modern supernova. However, a lesser appreciated fact about Brahe is that he extensively and systematically documented the trajectories of planets (#1).
In just a generation, along came Brahe's assistant, Johannes Kepler. Kepler deduced from all of Brahe's accurate observations that the trajectory of planets could be explained by three simple laws or regularities. First, by carefully making calculations of the Martian orbit, he noticed that all (known) planetary orbits were in fact elliptical, as opposed to the conventional Copernican belief before him that they were circular. He further noticed that the Sun was centered at one of the foci of the ellipses. Second, he noticed that the line joining the planet and the sun swept out sectors of equal areas along the orbit. Since orbits were elliptical, this implied that planets moved with variable speed---again a radical departure from Copernicus! He published both these observations in 1609 [I'm suprised he didn't write two papers on these potent mythbusters]. Third, he noticed that the square of the orbital period was proportional to the cube of the major axis of the elliptical orbit. He only published these 10 years later in 1619 [I wonder how he got tenure]. These are now known as Kepler's laws of planetary motion (#2).
Would Rutherford call Kepler a physicist? Would you call Kepler a physicist?
If we define a physicist narrowly as someone who engages in deductive reasoning based on observations of the natural world, then Kepler was not a physicist. However, if we recognize that a physicist must wear multiple hats along the journey from making observations (stamp collecting) to reasoning about them (physics a la Rutherford), then I would call him an exemplary physicist. Let's get more granluar about Kepler. What was he? Using modern terms, I would call him an applied statistician or more specifically, a curve fitter.
Kepler basically looked at the orbital trajectories and said, "wait a minute! this doesn't look circular to me. Hmmm...". He then selected a functional form to represent the trajectories (the equation of an ellipse) and simply fit the parameters to the data, the parameters being the foci and the major/minor axis of the ellipse to the orbit. Next, he looked carefully at the non-uniform speeds of the planet during the course of its revolution around the sun and said to himself, "Hmmm, what needs to be equal in order that the speeds can be unequal?". Finally he graphed the orbital period against the major axis length and mumbled, "There's a pattern here but I don't quite get it. Could it be a power law!". And it was.
In doing thus, Kelper had reduced the painstakingly detailed data recorded by Brahe into three simple regularities or laws. He made an epistemological innovation, i.e. he told us how to organize our observations neatly, in much the same way that Darwin organized species into the tree of life or Mendeleev organized the elements into a periodic table. Kepler's laws could now make accurate predictions about planetary motion.
In the parlance of modern statistics, we could interpret Kepler's laws as a descriptive statistical generative model. It described the statistics of planetary motion by generating them from underlying regularities. However, for all its genius, Kepler's work was merely descriptive, i.e. it succinctly answered the what questions but not the why questions. Why were planetary orbits elliptical? Why did they move faster when they came closer to the sun? For these answers, we had to wait another 100 years.
Newton and the why questions
Newton first formalized the concept of a force acting between two bodies. He postulated and verified the laws of motion. In posulating the gravitational force, and observing that the force was inversely proportional to squared distance, the universal theory of gravitation took shape.
Newton's theory was a causal explanation of the data observed by Brahe and the regularities captured by Kepler's laws: i.e. it could now answer the why questions. Just to take one example: as the planet comes closer to the sun, more force acts upon it, causing a greater acceleration increasing its speed!
I hazard a guess that Rutherford would have included Newton into his elite definition of a physicist!
Marr's three levels modified
From that prelude into the history of gravity, it is interesting to note that the answers to why questions also lead to how questions. How is gravitational force transmitted between two bodies without a medium? For nearly three centuries after Newton, a number of proposals were made for the mechanical explanation of gravity, all of which are known to be wrong today. The current explanation is attempted by quantum gravity, a theoretical framework that attempts to unify gravity with the other three fundamental forces, but as of today, we don't have a mechanistic explanation of gravity!
The sequence of what-why-how questions brings us to David Marr, a 20th century vision scientist and AI researcher, who postulated three necessary conditions for a computational theory of sensation or perception. Marr and his contemporaries conceived of vision as an information processing system. He said, to have a computational theory of a system, we need to understand:
- The computational level: what computation/ task does the system intend to perform?
- The algorithmic level: how does it represent this computation/ task and what strategies does it adopt to achieve its goal?
- The implementational level: what is the precise sequence of steps in the physical wet brain during the execution of the above algorithm?
Now, the universe is not an intentional system with a well defined goal, so Marr's postulates are more suited to building nature-inspired computational systems for solving specific tasks, rather than describing nature. Let's slightly modify (#3) Marr's levels to fit our what-why-how framework:
- What happens in the visual areas of the brain? [This is the stamp collecting task of Brahe]
- Can we build a simple statistical model that captures its regularities and predicts some of its dynamics? [This is the applied statistics / descriptive modeling task of Kepler]
- Why is it happening i.e. what is the brain trying to achieve through the observed dynamics? [This is the causal modeling task of Newton]
- How does it go about achieving its goal? [This is the mechanistic modeling task of quantum gravity]
With parables from Brahe down to Quantum Gravity, along with perspective from Marr, is it possible to meaningfully contextualize the need for theory in neuroscience as a discipline?
Let's take the primary visual cortex and see whether we can analyze developments about its understanding using the above framework.
The work of Brahe and (to a large extent) Kepler was done by the early greats (1960s onwards): David Hubel and Torston Wiesel. These guys measured from single neurons in the cat V1, recognized that there were cells selective to things like orientation, spatial frequency, ocular dominance, etc. [a Brahe task]. Next, along with Horace Barlow and other contemporaries, they explained natural images as constituted by oriented edges and gratings [a Kepler task]. Barlow and his contemporaries also attempted to give causal or normative explanations of what the visual cortex was doing. They proposed that the visual cortex was efficiently coding (in the Shannon information sense) the retinal image [a Newton task]. A little later, descriptive statistical generative models of natural images were proposed using Fourier and Gabor basis functions. The models were successful in describing the retinal image in terms of a few regularities [a Kepler task]. With the advent of artificial neural network models, it became possible to take the efficient coding hypothesis one step further and build mechanistic models of neural activity which efficiently represented the retinal image. It was possible to show mechanistically that efficient coding was realized by performing decorrelation in a distributed neural network to achieve this efficiency [a quantum gravity task]. With the advent of overcomplete basis functions: robustness, not just efficiency of visual information representation could also be normatively explained [a Newton task]. With further advances in natural image statistics by Olshausen and Field, it became clearer that besides efficient, decorrelated and robust coding of the retinal image, a key function of the visual cortex was to learn and update hypotheses (Bayesian posteriors) about the statistics of natural images [yet another Newton task]. How is Bayesian inference mechanically realized in a neural network? Again, artificial neural network models have been postulated for the same [yet another quantum gravity task].
One positive example does not make a theory, you quite rightly say (#4)? Ok. As we look around in other areas of neuroscience, what do we see?
I see that on a day to day basis we are all organized as cottage-industries and guilds, learning through apprenticeships, how to solve Brahe tasks, Kepler tasks, (and less frequently) Newton tasks, and quantum gravity tasks. Wider-scale databasing efforts [Brahe tasks] are also beginning to take place. Examples include Bert Sakmann's digital neuroanatomy project, the human connectome project (HCP), etc. Parallely, wider-scale big-data mining [Kepler tasks] is gaining ground. Examples include various connectomics projects, and contests to leverage big-data (such as the ADHD fMRI/ VBM/ DTI data analysis contest). Brahe and Kepler tasks certainly seem to be the mainstream activities of the day.
What do you see around you?
What would Feynman say to Rutherford?
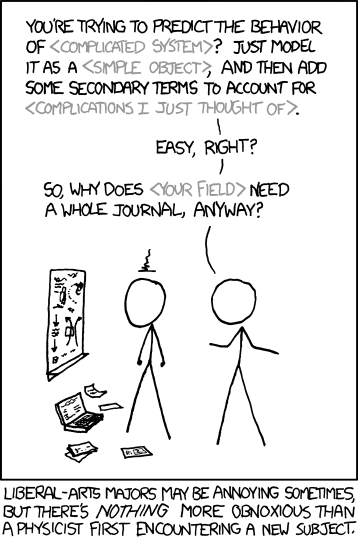
The above comic strip generated some brilliant discussion in the forum. A commentor succinctly summarized this to be the everlasting tension between Rutherford's famous quote and a pithy equivalent by Feynman, attributing the following quote to the latter:
Physicists often have the habit of taking the simplest explanation of any phenomenon and calling it physics, leaving the more complicated examples to other fields.
Is neuroscience ready for Newtons, or do we still need more Brahes and Keplers for now?
Here's another gem from the forum:
Physicists who do bad biology say, "It's so simple! Look, model it so." These do not understand the concept of 'unknown unknowns.' What if, for example, your insects normally behave predictably, but release an alarm pheromone when handled clumsily - for example, by a theoretical physicist?
Physicists who do good biology say, "It's so messy! Is there any way we can control for as much of that messiness as possible? How do we pry apart this noise to get at the underlying rules?" They bring their disdain for vague claims to the field, and back up their claims with data. They aren't airy anti-biologists, but intense, experiment-driven pragmatists.
Notes
(#1) Incidentally, Brahe was not the first to catalogue planetary motion. The Babylonians, the Greeks and the Chinese each built their own MySQL servers to document the movement of heavenly bodies across the sky, with the Chinese effort taking up the most servers by far.
(#2) Interestingly, laws do not come to be known as laws as soon as they are proposed. Voltaire was the first to refer to Kepler's observations as laws in 1738, more than 100 years after they were first published!
(#3) Here's a very interesting modification of Marr's levels discussing the difficulty of studying of hierarchical with emergent properties.
(#4) In a Feynman sense, you just got physicisted!
2 comments:
Does neuroscience need a Newton? Here's the answer: http://blogs.scientificamerican.com/scicurious-brain/files/2012/12/Screen-shot-2012-12-03-at-1.31.42-PM.png
Haha!
Post a Comment